Our guest today is Maggie Murphy, Associate Professor in the University Libraries and Art & Design Librarian. Our conversation today focuses on the intersection of EDI and Artificial Intelligence.
Hosted by: Brad Johnson and Nodia Mena
Intro Music, The Garifuna Collective, Weyu Larigi Weyu
Outro Music, A Short Walk, from Zapsplat.com,
Quote from the Episode
“AI is a concept that has been around since about the 1950s. And it refers to a machine’s ability to perform a task that would have previously required human intelligence. A lot of recent applications of AI have been a form of AI called generative AI. That’s the ability of AI programs and systems to create something new, like an image or a song or a text. Just as we can create, we can learn to create things like art or music, by studying patterns that we perceive across many examples of existing works. Generative AI uses data to learn those same patterns and create content on its own, through a process called deep learning, which is modeled on how the human brain works.”
(This transcript was generated by AI with some human edits.)
Welcome to small steps big impact, a podcast conversation about equity, diversity and inclusion in our classrooms. So today on our podcast, there are actually two of us who are hosting and speaking with us with our guests. So, my name is Brad Johnson, he him his pronouns. I’m a Clinical Professor of higher education over in the School of Education.
Hi, I am Nodia Mena, and I am a doctoral student in the School of Education.
Our guest today is Maggie Murphy, associate professor in the University Libraries and our Design librarian. Our conversation today will focus on the intersection of EDI and artificial intelligence. Thank you for joining us, Maggie. Today we’re talking about artificial intelligence or commonly known as AI, and visual imagery and art. But why don’t we start out with you kind of giving us a brief overview of what artificial intelligence AI is?
Okay, I can definitely do that. So, AI is a concept that has been around since about the 1950s. And it refers to a machine’s ability to perform a task that would have previously required human intelligence. So that’s the intelligence part. A lot of recent applications of AI have been a form of AI called generative AI. So that’s the ability of AI programs and systems to create something new, like an image or a song or a text. So just as we can create, we can learn to create things like art or music, by studying patterns that we perceive across many examples of existing works. Generative AI uses data to learn those same patterns and create content on its own, through a process called Deep Learning, which is modeled on how the human brain works. So, it uses many layers of neural networks, which are modeled in the human brain to recognize patterns and make connections between data in order to mimic them and create new things.
Thank you so much for that answer. Maggie, that was great. And thank you also for making clarifications, you know, in regards to some of the assumptions that are made in regards to artificial intelligence, and in the spirit of making those and expanding on those qualifications, can you share a little about your research and experience studying using artificial intelligence with students in higher education?
Sure. So as the Art and Design Librarian, I work a lot with students in the School of Art, not just those who are engaging in traditional scholarship in art history, or the humanities, and writing papers, but students whose primary scholarly output is the creation of new artworks, studio art students, new media and design are some of our concentrations animation. I think a lot about their relationship to information and research and scholarship as creators. And in addition, I’m a practicing artist, and I, you know, have that kind of personal interest, the relationship between technology and art, the nature of creativity. And so artificial intelligence raises a lot of interesting questions in those areas. And even before mid 2022 is when we started to see the newer image generation tools, Dolly two, which is an image generation tool, from open AI, which is the same company that has made ChatGPT available. Their image generation tools, Dall-e two, opened a public research preview of their second version of the model in mid 2022, and shortly after that, it was a big leap forward from their initial version of Dolly, which is Dall-E. But as a play on the artist, Salvador Dali. Mid journey and stable diffusion are two other companies that opened their models to public usage and there’s just been an explosion since then. But prior to that, there have been other image generation tools that required some level of computer coding to get work. They didn’t have a GUI or graphical user interface. Okay, so you kind of you know, there wasn’t a form where you entered in text and it shot out an image, you had to do some coding in Python Jupiter Notebook. And these were kind of more rudimentary tools. One was a model called V. Q, Gann and clip. So again, is a generative adversarial network that kind of pits two neural nets against each other who are trying to create an image that is more realistic or relevant to the prompt. And clip is the text to image part of the model. So all of the models now you enter text input, and it tries to output an image that meets the prompt. That was the case earlier, but it wasn’t as easy as just entering your prompt. And so I had been playing around with that already, because I was interested in it. And once the sort of wider availability of these tools kind of exploded when mid 2022 became clear to me that similar to chat GPT, and the large language model tools that are now available to students, this was going to be something that would impact kind of wider visual culture, media literacy, things like that, outside of just the interest of artists who want to play with technology. And so that’s kind of where I, you know, got to the current point is that I, I realized that I needed to know about it for the sake of working with my students.
So speaking of students in classrooms, how can AI generated visuals be utilized in teaching and learning by faculty working with their students?
Sure. There are a couple of different ways to approach that. The first is that the AI tools can generate new visuals used by faculty, in their teaching materials and presentations, both as simple illustrations, but also as kind of speculative images that might envision something that wouldn’t necessarily be possible, like a historical event, or something like that, as a discussion prompt for students, because the tools are really able to construct realistic images, incorporate images of people who exist in the world. So much so that in recent kind of new cycles, there have been images that have been mistaken as being real, like an image of the Pope wearing a kind of cool streetwear puffer jacket gets abstracted from the context of somebody who generated it. And said, AI made this, you know, and just gets reshared, to the point where people are seeing it, and they think it is, you know, a photograph by a news agency. And so that is kind of using that, in a way to generate things, for students to think about critically and discuss is one way that faculty can make use of it. Another is simply because the visual, or the interaction of visuals, and copyright is really complicated. And there’s a lot of misunderstandings about how copyright law applies to visuals, the idea that if you can access something for free online, it also is copyright free, is a wide perception about images and copyright, among students amongst faculty amongst, you know, the public. And so, we’ve worked really hard to encourage the use of open access images, images from the public domain for which copyright has expired, or doesn’t apply for various reasons, including that they were created by the federal government. That’s one of the exceptions to copyright law. So images by like NASA, of the beautiful like, you know, telescope images of space, those are already in the public domain. But then images shared under a Creative Commons or other open license framework. So AI could be an alternative to those in that you can generate an image that hadn’t previously existed for use, you know, in your presentations, etc. And another way would be to look at the technology itself and the process by which it creates new images, which would involve getting to know more about it. And using that as a sort of model for students about the creation and editorial process us thinking about the nature of creativity, and also the nature of intellectual property and our place in ongoing discussions and the kind of history of, of visual culture. A lot of the issues with track GPT. And, you know, citation, there’s the issue that students may not attribute the output to a tool. But the kind of broader issue is that those ideas that are output in chat GPT come from somewhere. And they aren’t necessarily attributed to those thinkers, those scholars, etc, by the software, and the student doesn’t necessarily know any better, because they don’t have the expert level knowledge, the faculty member does the same thing. With output of these tools, you know, that someone who studies art might be able to look at these formalistic elements, shapes, colors, composition, and say, Oh, that’s clearly, you know, derivative of this art style or something like that, that a student necessarily might or might not necessarily know. And so kind of breaking down the components and thinking about how these tools did work to the point where they can create something, quote, unquote, new, but it still has to come from somewhere. And so thinking about, you know, what is it mimicking? How does it create new things? What does that mean, in terms of human creativity is AI, a tool, a new medium, a co-creator. And so I think that has an application, you know, in communication studies and Media Studies, and public history. A lot of different disciplines outside of Art and Design,
You touch on very, very important points. And just to kind of expand on what you just said, what are some ethical issues or concerns that you have researched or are aware of related to AI generated images and visuals. One of the big ones is a kind of more recent trend towards generating images, of people of color of people of different gender, gender representations of people who have physical disabilities, by clothing companies, magazines, instead of hiring models, who so in a way, it can increase the representation that people see. But in another way, it just perpetuates a lot of issues, in media industries. And it also AI just like, all systems created by people can reinforce existing stereotypes and biases. And we’ve seen that in AI, that make decisions about who gets a credit card. Even as simple as search engines, when you, you know, ask for an image of a scientist, and it’s all white men, you know, you ask for an image of, I’m trying to think of a professor, you know, and it’s all stereotypical, you know, old white men, quarter a coat with the, you know, elbow patches, etc. That same, those same biases are replicated in the generation technology too. So if you ask for a scientist, it will probably generate, you know, an image of a white man in a in a coat. And so these issues are, you know, people are aware of them. And the these are private companies, you know, who they have to think about, are they do think about who’s using the tool for what, you know, they’re thinking about the marketability, etc. And I’m not sure that issues of visual BIAs are at the top of their concern necessarily, but it has been something that has been noted and will probably continue to be an issue so long as they are using datasets that have these biases embedded. However, on the flip side, that technology does allow people to create new representations and new visions for what they want to see in the world. So, in the list of resources that I’ve shared that could be you know, shared with this podcast episode, there was an article from Buzzfeed News or Earlier this year, were talking about artists are using AI to create fat, black sci fi and fantasy characters, you know, characters and images, you know of genres and ideas that artists, commercial artists maybe have not created, you know, and people are able to create images in their own in their own image or in their own ideas, using these tools, some other ethical images, ethical issues, some other ethical issues have to do with the way that the tools use data that are based on other people’s copyrighted works of intellectual property without their explicit permission. So when you use a tool that generates images, most of them use data that has been scraped from the internet, including images that are created by artists who have not kind of consented to the use of their image in that way. And this is kind of a gray area in copyright, there are lawsuits pending against a lot of the large AI companies like mid journey, stable diffusion, open AI on this issue. But just as you as an individual would be liable for using an image without that person’s permission, especially for commercial applications, if it didn’t qualify for a fair use carve out and copyright law, these, these companies are using them on a way higher level, and to the point where the artists are able to demonstrate how you can use their name and a prompt to create an image in their recognizable style. And so it’s, it’s creating a way for you to kind of subvert their intellectual property, especially in these commercial applications. Like generating, you know, kind of things that you would hire an illustrator for, or a comic artist or something like that. And so it’s not necessarily something that people think about when they use the tool, but every time you generate an image, you’re kind of engaging with a technology that uses a model that is kind of at its core. Ethics ethically, you know, squeaky, I don’t know the term to use there. That’s another level of plagiarism it is it is another level of plagiarism. And then there are issues with all of the large language models and tools that use them. Because even the image generation tools do require language data in that you have to take the text and convert it to image. So that involves labeling of images with text, and that is something that is done manually. Some datasets, they’re able to scrape labels with the images. From captions, you know, from metadata embedded in the image, but there’s a lot of human labeling. I think it was open ai ai, and I would have to double check on that. There were articles about their employees and Kenya getting paid like $2 a day to label data. So there are labor issues. The tools themselves are quite resource intensive, not perhaps at the level of Bitcoin mining, but the tools require a lot of energy, water cooling, for the servers, things like that, that, you know, contribute to the climate crisis. And so even though we think of it as just something in the cloud, and you know, we press a button on a computer, all of these systems, you know, exist in the world and have real impact with people. And I want students to know about that, so that they can engage in informed decision making around the use of these tools. And think about not only how it impacts other people who have created images, but potentially their own work and their images that they share on Instagram things that they post online on their own websites, these are being scraped as well. And some of them may contribute to things like facial recognition technologies, you know, that monitor people. And so I think we really need to come together as a society and think about AI applications beyond the cool new things that they can do for us and think about how we are contributing to them passively without kind of fighting back against, and that’s what a lot of the lawsuits are doing. But also just demanding transparency from these companies about how their systems work, and what the broader implications of them beyond just the creation of new images, what else is happening?
I wanted to follow up, I don’t know if you had seen this article, but there was a self-identified Asian American woman. And she had a picture of herself. And she uploaded it into one of these visual AI generators, and said, basically make this professional hits. She was trying to, I think, update her LinkedIn profile or something. The image that came back, turned her into a white woman with blue eyes. And I think different color hair, different texture hair, what do you I mean, I know you talked about that, that AI and the visuals are all built on, you know, scraping things, and but it was just amazing to me that it would come back.
There are now a whole bunch of tools having to do with headshots and, and images. And so it could be that that particular tool really only had white women in its data set, you know, and so the process that it uses, involves a technique called diffusion, in which an image a bunch of like, visual noise is added to it static, and then it tries to decrypt the static back into an image. And in that process, if there are only white women in the data set, when it decrypts, it, it’s going to fill back in the eyes with, you know, a white woman’s eyes and with, you know, Eurocentric hair texture and things like that. And so that shows the kind of limitations of the tools based on the data that they are using, and especially a company that is building its own tool, with limited data sets. And not necessarily if it is, you know, white people running the company, then they may think these are what people look like, you know, and our tool should function as we see fit, because we’ve only tested it against white people. And that is probably what happened. Sorry, that is probably what happened in this case. But we’re seeing that even in the larger tools that use giant datasets, especially the you know, coded words, like professional, you know, which can be, you know, in, in larger discourses we know, are associated with, you know, middle class people, white people have been used to, you know, kind of police people of color, queer people in, in employment settings, you know, that is just kind of reified by the technology.
One of the things that really fascinated me about your work is part of the tenets for learning visual literacy, which is how learners can pursue social justice through visual practices. What you just say right now is highly related to the ways in which learning about visual literacy can anticipate whether algorithms perpetuate or helped to deconstruct existing social norms that generate power imbalances. Now, my question is, has there been any challenges to the work that’s been done to deconstruct these issues in society, in the work that you’re doing?
What you’re referring to is a document that I worked on with a group of people called the framework for visual literacy in higher education. And it’s a framework that has been was created under the kind of auspices of but has been adopted by the Association of college and research libraries, which is the branch of the American Library Association is the major professional organization for librarians who work in higher education. And so it’s a teaching and learning document that speaks to what we hope students should be able to do with visual information of all kinds. And so there are four themes, to the framework for visual literacy, and the pursuit of social justice through visual practice is one of them. And the three other themes, they all have associated knowledge practices, what students should be able to do, and dispositions. And those are reflective or metacognitive, practices, things that they should be able to think about, and understand. And each of the themes, associated knowledge, practices and dispositions. The ones having to do with social justice then appear in the social justice theme, because we wanted to emphasize that social justice is not something separate. But it’s something deeply integrated into all of these different, you know, ways of interacting with information. And so the sort of critical look at algorithms in visual culture is part of that. Frame, but so are things like, thinking about the accessibility of visuals that you create, and that actually is an interesting intersection with AI. In that, we’ll see a lot of AI tools to generate alt text for images, and the ability, you know, are we thinking critically about whether not only is it accurate, but is it useful for people who use accessibility tools for digital visuals? does it describe what they need to know about the image and not just you know, the image of a cat or a person, you know, lacking all of the context and things like that, because it becomes a shortcut, just like automatically generated captions or transcripts for video, you know, video. Something, people just post those, you know, unedited. Also, things like in the process of visual creation, giving credit to others, not kind of using or abstracting imagery that comes from communities and cultures other than your own, in a way that, you know, harms the original creators, but also closed communities, you know, whose imagery is culturally important or sacred. And that is something that potentially AI does. And again, students may not even realize, you know, that this motif is deeply associated with, you know, Pacific Northwest indigenous people, you know, when they asked for, you know, kind of a geometric image of a bird or something, and what it’s referencing is something specific. And so that is why we wrote the document that way, in that every time you create something, or engage with something existing, you are interacting with systems of power, you know, representation. Like money, you know, paying creators is part of it, that you’re not using things for free, where the creators have asked for compensation, things like that. And so when it has to do with algorithms, thinking also about the systems, what they’re showing you what versus what you’re not being shown, algorithms play into what are called recommender systems, which power the recommendations you get on Spotify or Netflix, you know, you might like X, Y, and Z. Also, your tick tock, you know, for you page, your Instagram discovery feed. What you see can be based on what you already interact with. And for in the context of art students I think about that a lot in that they’re not necessarily being exposed to even outside of thinking about EDI media and, you know, composition and things. They put themselves in a box very early into their creative education. If they only like, you know, like realistic tat to our you know, that’s they’re just going to keep seeing more of it, you know, and we think of analog ways to break them out of the box browsing through books in the library, things like that. But those same systems can create a filter bubble, you know, so that you are not learning about other points of view, other ways of engaging with the world, other knowledge practices. And so that is part of the critical thinking about algorithms and social justice and visual practice.
Nodia and I tried to ask our guests some some kind of takeaways that we can provide our our listeners. What suggestions would you give to faculty who may want to explore a little bit more about air generated images and visuals, but really don’t have the knowledge of where to start with this new technology? Sure. The first thing I would say is to try them out, because I think that until you do, you may be surprised by what they are capable of at this point and the extent to which they are rapidly evolving in their capabilities. Just a year ago, you know, there was a lot of discourse around, oh, you can tell something’s air generated because the people’s hands have too many or too few fingers or something like that. And as an aside, I think that’s a problematic model anyway, because it places normative expectations on bodies, you know, where you’re putting people’s bodies under, you know, surveillance and people who do not, you know, have five fingers on their hands. You know, it’s like that. It’s just a picture of them and not necessarily something they generate. But anyway, you know, all this sort of tells that you’re supposed to be able to tell it’s an AI generated image. Those are rapidly becoming outmoded in that the technology, because it is a machine learning technology, which means that it learns from the interaction with users and from the data.
And they are becoming just kind of mind blowing in their capability to take a description and generate an image in a range of styles, things like you might say, security footage of, you know, something you know, happening. And it will do it in the style of, you know, a security camera or, you know, things like that. And so the ability to just put out into the world, you know, images that trick our brains, you know, in a way that we’re not prepared for that are persuasive to our brains because we have for a large, you know. Percentage, you know of of time with photographic images.
Been able to more or less trust what we see and so play around with technology and then think about where it intersects with your discipline. For example, this is separate, but you know, things like 3D printing, you know, it’s not just about being able to create like fun little toys,
but faculty and archeology are able to print things that people have recently dug up across the world and modeled, you know, so that the object is in their classroom. Students who are taking health science classes can, you know, interact with models of things inside the human body or, you know, bones, teeth, things like that. Virtual reality is used a lot again in health science settings because you’re allowed you can put the student in a patient interaction setting or a care setting, you know, that is modeled for in virtual reality. And so thinking about those similar applications outside of, you know, the kind of art and design setting for image generation students can generate political cartoons that comment on current events. They can generate things for storytelling, you know, for rhetorical analysis. Potentially look at the difference between what the tool generates, you know, around a scientific image versus what, you know, we are able to discern with a telescope or something like that. As a critical thinking exercise, as a deep looking exercise. And then one of the sort of major things that I like about technology is that it models a Taking feedback and refining the output in a way that I think will resonate with students in that the sort of drafting process of writing, creating process of visuals, you know, of preparing speech and so on is not just a linear process in which you get your assignment, you create your final product, you know, you get graded but should be, you know, iterative. As you get feedback from a faculty member, you know, you adjust the process and the tools do that in real time and you can see the way that they do that. I think that that is an interesting thing to get students to think about, about their own learning process and their own, you know, process of creating scholarship, of creating visuals and so on.
I am so fascinated. Thank you so much for sharing so much knowledge with us. Amazing. Thank you. I appreciate it. Thank you, Maggie. Thanks for having me. Thank you for joining us today on small steps. Big impact. Our goal is to have continuous conversations about equity, diversity and inclusion in our classrooms. To learn more about EDI, please go to our website. Feel free to leave us suggestions for future topics and resources or join me on the episode for a conversation.
About our Guest

Maggie Murphy
Maggie Murphy is the Art & Design Librarian and associate professor in the UNC Greensboro University Libraries. She works with students, faculty, and curators in the Schools of Art, Theatre, and Dance, the departments of History, Interior Architecture, Media Studies, Philosophy, and Religious Studies, and the Weatherspoon Art Museum towards the goal of ethical and critical information access, use, and creation.
Maggie is a co-author of the ACRL Framework for Visual Literacy in Higher Education and the lead editor for Unframing the Visual: Visual Literacy Pedagogy for Academic Libraries and Information Spaces (forthcoming from ACRL Press). Her scholarly interests include practice-based research methods for studio artists and speculative pedagogies for creative engagement with AI. She is a printmaker and mixed-media artist.
References from the Episode
- Harnessing Pandora’s Box: At the Intersection of Information Literacy and AI [Lesson plans from librarians at Miami University, OH]
- “Humans Are Biased, Generative AI Is Even Worse,” Bloomberg, September 19, 2023
- “Using AI to Protect Against AI Image Manipulation,” MIT News, July 31, 2023
- “The Ethics of Making (and Publishing) AI Art,” Lifehacker, July 31, 2023
- “Science & Tech Spotlight: Generative AI,” U.S. Government Accountability Office, June 13, 2023.
- “Will AI-Generated Images Create a New Crisis for Fact-Checkers? Experts Are Not So Sure,” Reuters Institute/University of Oxford, April 11, 2023
- “Levi’s Announced They’d Use AI-Generated Models to ‘Increase Diversity.’ Black Models Say It’s a Step Backward and They Should Book Real People Instead,” Insider, April 4, 2023
- “‘This Is What Your Worlds Are Lacking’: Artists Are Using AI To Create Fat, Black Sci-Fi and Fantasy Characters,” Buzzfeed News, February 25, 2023
- “This Is Not a Bicycle: Human Creativity and Generative AI,” Creative Commons, February 21, 2023
- “The Text-to-Image Revolution, Explained,” Vox, June 1, 2022
Brad and Nodia asked Maggie to generate AI-generated images based on brief prompts as a sample of what AI-generated images look like.
Brad gave the prompt of an image of what a diverse student body looks like on a college campus. Here are the images that were generated:


Nodia gave the prompt of an image of Garifuna people. Here are the images that were generated:
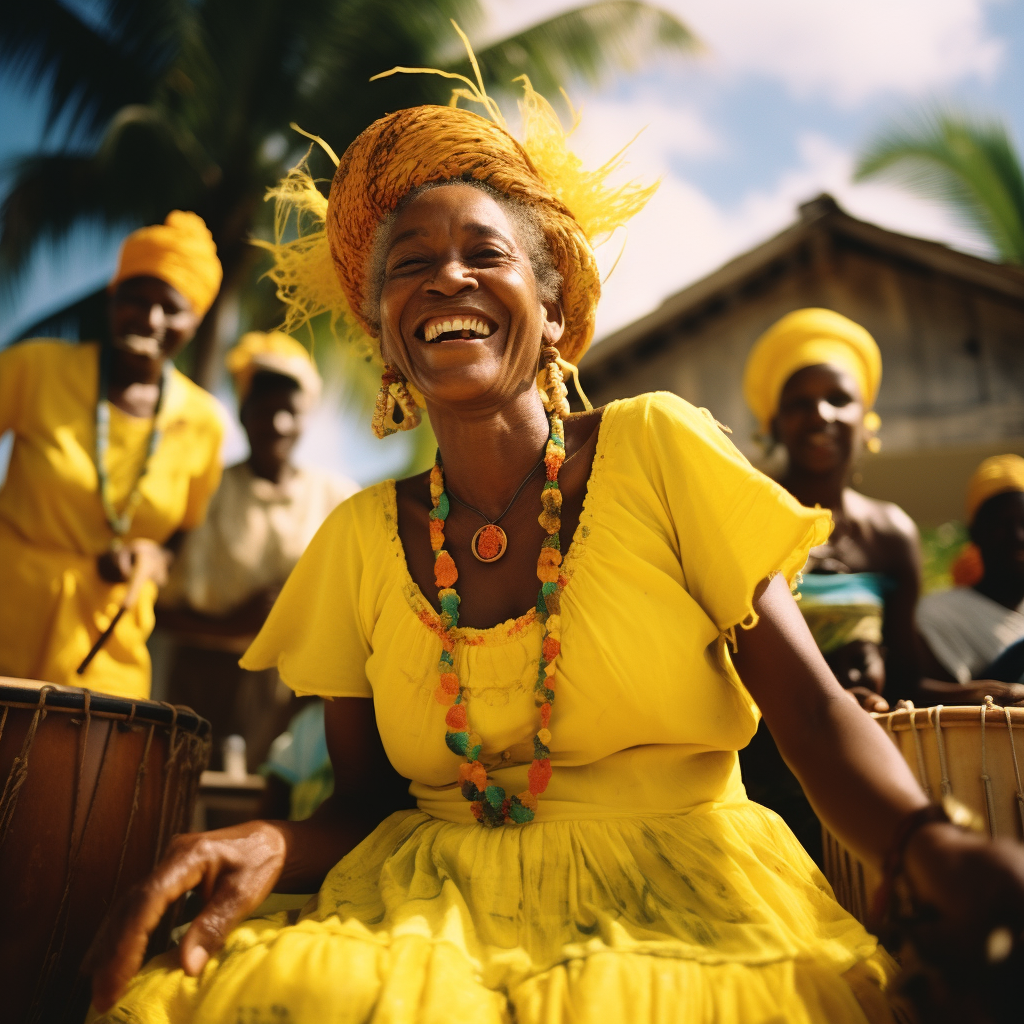
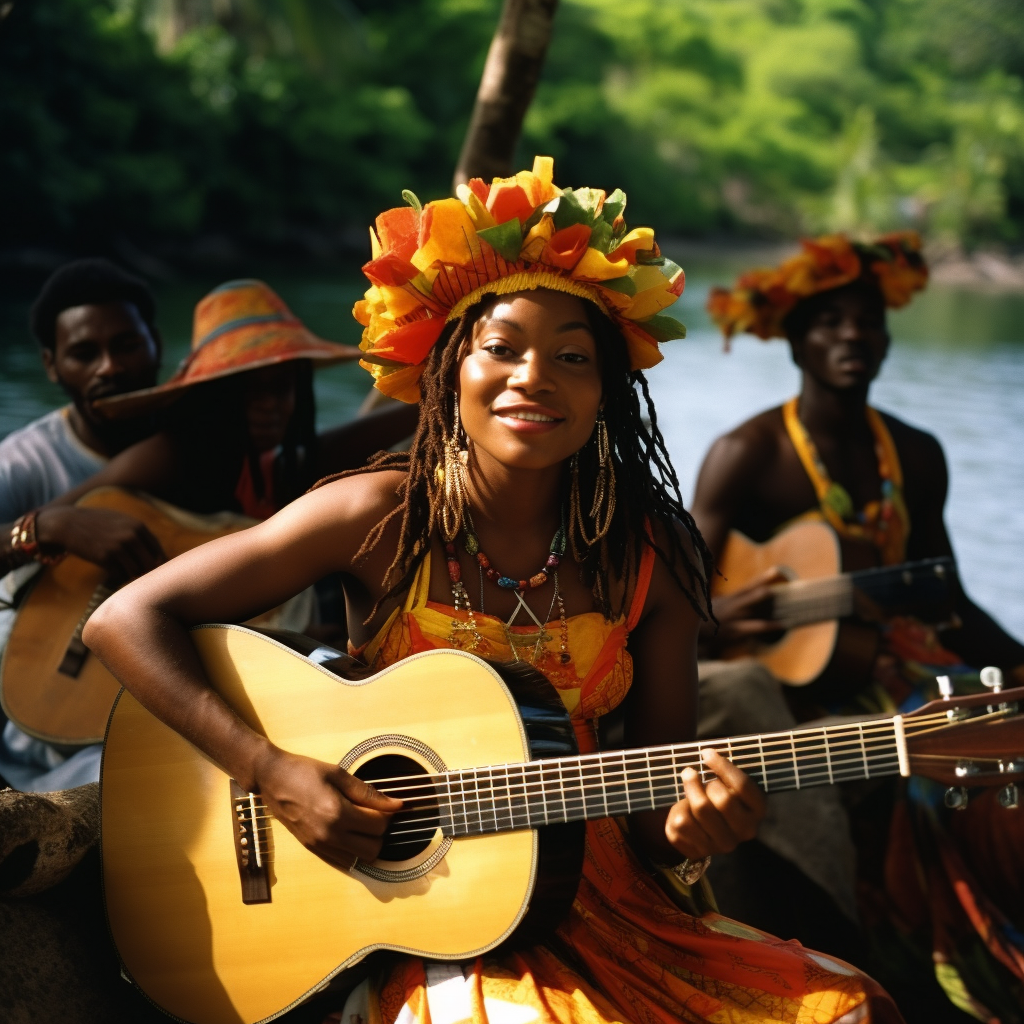